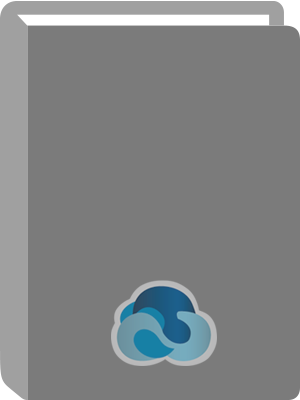
Robot learning from human teachers
INITIAL_TITLE_SRCH:
Robot learning from human teachers
AUTHOR:
Chernova, Sonia.
Thomaz, Andrea L.
ISBN:
9781627051996
PUBLICATION_INFO:
[San Rafael, California] : Morgan & Claypool, c2014.
PHYSICAL_DESC:
xi, 109 p. : ill. ; 23 cm
SERIES:
Synthesis lectures on artificial intelligence and machine learning ; #28
Synthesis lectures on artificial intelligence and machine learning ; #28.
SERIES_TITLE:
Synthesis lectures on artificial intelligence and machine learning ;
GENERAL_NOTE:
Part of: Synthesis digital library of engineering and computer science.
ABSTRACT:
Learning from Demonstration (LfD) explores techniques for learning a task policy from examples provided by a human teacher. The field of LfD has grown into an extensive body of literature over the past 30 years, with a wide variety of approaches for encoding human demonstrations and modeling skills and tasks. Additionally, we have recently seen a focus on gathering data from nonexpert human teachers (i.e., domain experts but not robotics experts). In this book, we provide an introduction to the field with a focus on the unique technical challenges associated with designing robots that learn from naive human teachers. We begin, in the introduction, with a unification of the various terminology seen in the literature as well as an outline of the design choices one has in designing an LfD system. Chapter 2 gives a brief survey of the psychology literature that provides insights from human social learning that are relevant to designing robotic social learners. Chapter 3 walks through an LfD interaction, surveying the design choices one makes and state of the art approaches in prior work. First, is the choice of input, how the human teacher interacts with the robot to provide demonstrations. Next, is the choice of modeling technique. Currently, there is a dichotomy in the field between approaches that model low-level motor skills and those that model high-level tasks composed of primitive actions. We devote a chapter to each of these. Chapter 7 is devoted to interactive and active learning approaches that allow the robot to refine an existing task model. And finally, Chapter 8 provides best practices for evaluation of LfD systems, with a focus on how to approach experiments with human subjects in this domain.
1. Introduction -- 1.1 Machine learning for end-users -- 1.2 The learning from demonstration pipeline -- 1.3 A note on terminology. 2. Human social learning -- 2.1 Learning is a part of all activity -- 2.2 Teachers scaffold the learning process -- 2.2.1 Attention direction -- 2.2.2 Dynamic scaffolding -- 2.2.3 Externalizing and modeling metacognition -- 2.3 Role of communication in social learning -- 2.3.1 Expression provides feedback to guide a teacher -- 2.3.2 Asking questions -- 2.4 Implications for the design of robot learners. 3. Modes of interaction with a teacher -- 3.1 The correspondence problem -- 3.2 Learning by doing -- 3.3 Learning from observation -- 3.4 Learning from critique -- 3.5 Design implications. 4. Learning low-level motion trajectories -- 4.1 State spaces for motion learning -- 4.2 Modeling an action with dynamic movement primitives -- 4.3 Modeling action with probabilistic models -- 4.4 Techniques for handling suboptimal demonstrations. 5. Learning high-level tasks -- 5.1 State spaces for high-level learning -- 5.2 Learning a mapping function -- 5.3 Learning a task plan -- 5.4 Learning task objectives -- 5.5 Learning task features -- 5.6 Learning frame of reference -- 5.7 Learning object affordances -- 5.8 Techniques for handling suboptimal demonstrations -- 5.9 Discussion and open challenges. 6. Refining a learned task -- 6.1 Batch vs. incremental learning -- 6.2 Reinforcement learning based methods -- 6.3 Corrective refinement from the teacher -- 6.4 Active learning -- 6.4.1 Label queries -- 6.4.2 Demonstration queries -- 6.4.3 Feature queries -- 6.5 Summary. 7. Designing and evaluating an LfD study -- 7.1 Experimental design -- 7.2 Evaluating the algorithmic performance -- 7.3 Evaluating the interaction -- 7.3.1 Subjective measures -- 7.3.2 Objective measures -- 7.4 Experimental controls -- 7.5 Experimental protocol -- 7.6 Data analysis -- 7.6.1 Choosing the right statistical tool -- 7.6.2 Drawing conclusions -- 7.7 Additional resources. 8. Future challenges and opportunities -- 8.1 Real users, real tasks -- 8.2 HRI considerations -- 8.3 Advancing learning through benchmarking and integration -- 8.4 Opportunities -- 8.5 Additional resources. Bibliography -- Authors' biographies.
SUBJECT:
Robots -- Control systems. |
Machine learning. |
Human-robot interaction. |
BIBSUMMARY:
Learning from Demonstration (LfD) explores techniques for learning a task policy from examples provided by a human teacher. The field of LfD has grown into an extensive body of literature over the past 30 years, with a wide variety of approaches for encoding human demonstrations and modeling skills and tasks. Additionally, we have recently seen a focus on gathering data from nonexpert human teachers (i.e., domain experts but not robotics experts). In this book, we provide an introduction to the field with a focus on the unique technical challenges associated with designing robots that learn from naive human teachers. We begin, in the introduction, with a unification of the various terminology seen in the literature as well as an outline of the design choices one has in designing an LfD system. Chapter 2 gives a brief survey of the psychology literature that provides insights from human social learning that are relevant to designing robotic social learners. Chapter 3 walks through an LfD interaction, surveying the design choices one makes and state of the art approaches in prior work. First, is the choice of input, how the human teacher interacts with the robot to provide demonstrations. Next, is the choice of modeling technique. Currently, there is a dichotomy in the field between approaches that model low-level motor skills and those that model high-level tasks composed of primitive actions. We devote a chapter to each of these. Chapter 7 is devoted to interactive and active learning approaches that allow the robot to refine an existing task model. And finally, Chapter 8 provides best practices for evaluation of LfD systems, with a focus on how to approach experiments with human subjects in this domain.
1. Introduction -- 1.1 Machine learning for end-users -- 1.2 The learning from demonstration pipeline -- 1.3 A note on terminology. 2. Human social learning -- 2.1 Learning is a part of all activity -- 2.2 Teachers scaffold the learning process -- 2.2.1 Attention direction -- 2.2.2 Dynamic scaffolding -- 2.2.3 Externalizing and modeling metacognition -- 2.3 Role of communication in social learning -- 2.3.1 Expression provides feedback to guide a teacher -- 2.3.2 Asking questions -- 2.4 Implications for the design of robot learners. 3. Modes of interaction with a teacher -- 3.1 The correspondence problem -- 3.2 Learning by doing -- 3.3 Learning from observation -- 3.4 Learning from critique -- 3.5 Design implications. 4. Learning low-level motion trajectories -- 4.1 State spaces for motion learning -- 4.2 Modeling an action with dynamic movement primitives -- 4.3 Modeling action with probabilistic models -- 4.4 Techniques for handling suboptimal demonstrations. 5. Learning high-level tasks -- 5.1 State spaces for high-level learning -- 5.2 Learning a mapping function -- 5.3 Learning a task plan -- 5.4 Learning task objectives -- 5.5 Learning task features -- 5.6 Learning frame of reference -- 5.7 Learning object affordances -- 5.8 Techniques for handling suboptimal demonstrations -- 5.9 Discussion and open challenges. 6. Refining a learned task -- 6.1 Batch vs. incremental learning -- 6.2 Reinforcement learning based methods -- 6.3 Corrective refinement from the teacher -- 6.4 Active learning -- 6.4.1 Label queries -- 6.4.2 Demonstration queries -- 6.4.3 Feature queries -- 6.5 Summary. 7. Designing and evaluating an LfD study -- 7.1 Experimental design -- 7.2 Evaluating the algorithmic performance -- 7.3 Evaluating the interaction -- 7.3.1 Subjective measures -- 7.3.2 Objective measures -- 7.4 Experimental controls -- 7.5 Experimental protocol -- 7.6 Data analysis -- 7.6.1 Choosing the right statistical tool -- 7.6.2 Drawing conclusions -- 7.7 Additional resources. 8. Future challenges and opportunities -- 8.1 Real users, real tasks -- 8.2 HRI considerations -- 8.3 Advancing learning through benchmarking and integration -- 8.4 Opportunities -- 8.5 Additional resources. Bibliography -- Authors' biographies.